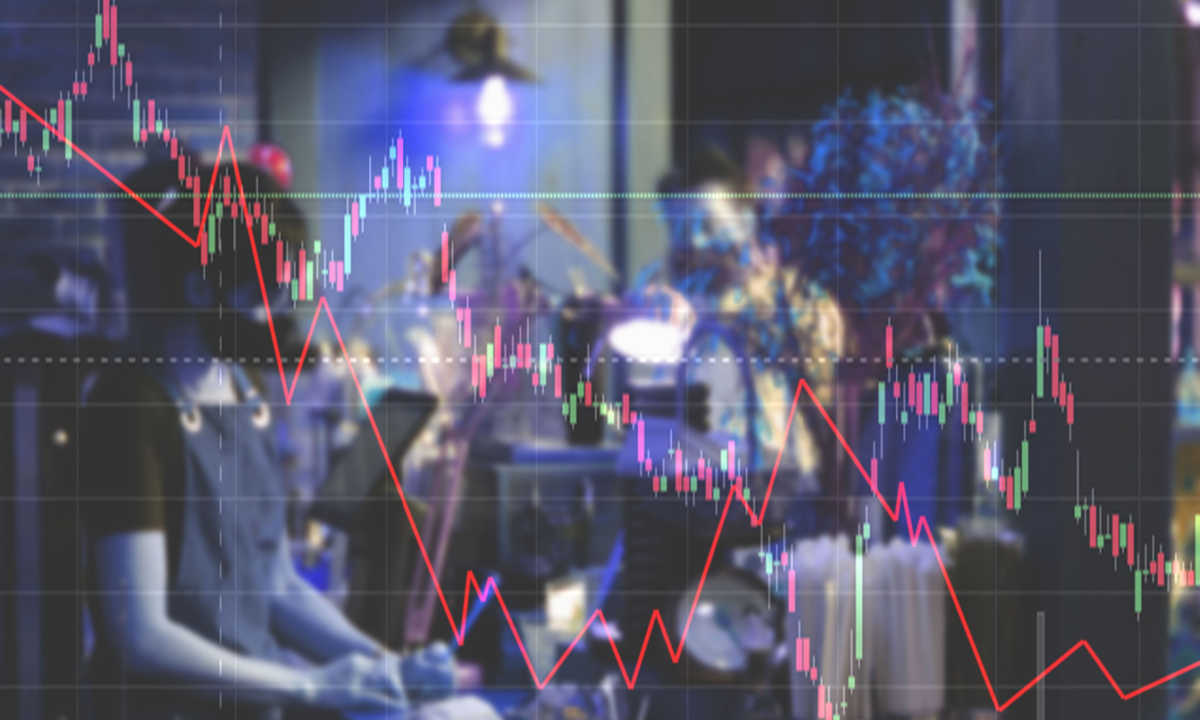
By: Ronald Crelinsten (Center for International Governance Innovation)
As with any emerging technology, generative artificial intelligence (AI) models present a dual nature. They offer innovative solutions to existing problems and challenges, but they can also enable harmful activities such as revenge porn, sextortion, disinformation, discrimination, and violent extremism. Worries have grown regarding AI “going rogue” or being misused in inappropriate or unethical manners. As aptly noted by Marie Lamensch, “generative AI creates images, text, audio, and video based on word prompts,” thereby exerting a wide-ranging influence on digital content.
Stochastic Parrots
The term “stochastic parrot” was coined by Emily M. Bender, Timnit Gebru, and their colleagues to describe AI language models (LMs). They defined an LM as a system that haphazardly strings together sequences of linguistic forms observed in extensive training data, guided by probabilistic information on their combinations, yet devoid of any understanding of meaning. These models essentially mimic statistical patterns gleaned from large datasets rather than comprehending the language they process.
Bender and her team shed light on some detrimental consequences, pointing out that prevailing practices in acquiring, processing, and filtering training data inadvertently favor dominant viewpoints. By considering vast amounts of web text as universally representative, there’s a risk of perpetuating power imbalances and reinforcing inequality. Large LMs can generate copious amounts of coherent text on demand, allowing malicious actors to exploit this fluency and coherence to deceive individuals into perceiving the content as “truthful.” Lamensch contends that without appropriate filters and mitigation strategies, generative AI tools absorb and replicate flawed, sometimes unethical, data.
In specific terms, generative AI models are trained on a limited dataset often rife with misogyny, racism, homophobia, and a male-centric perspective. A persistent gender gap exists in internet and digital tool usage, as well as in digital skills, with women less likely to engage with such tools or develop related skills, particularly in less developed countries. Women who do participate online often become targets of sexualized online abuse more frequently than men. This represents the darker facet of AI, whereby generative AI models reinforce harmful stereotypes, mirroring the biases and ideologies embedded in their source material…
Featured News
Big Tech Braces for Potential Changes Under a Second Trump Presidency
Nov 6, 2024 by
CPI
Trump’s Potential Shift in US Antitrust Policy Raises Questions for Big Tech and Mergers
Nov 6, 2024 by
CPI
EU Set to Fine Apple in First Major Enforcement of Digital Markets Act
Nov 5, 2024 by
CPI
Six Indicted in Federal Bid-Rigging Schemes Involving Government IT Contracts
Nov 5, 2024 by
CPI
Ireland Secures First €3 Billion Apple Tax Payment, Boosting Exchequer Funds
Nov 5, 2024 by
CPI
Antitrust Mix by CPI
Antitrust Chronicle® – Remedies Revisited
Oct 30, 2024 by
CPI
Fixing the Fix: Updating Policy on Merger Remedies
Oct 30, 2024 by
CPI
Methodology Matters: The 2017 FTC Remedies Study
Oct 30, 2024 by
CPI
U.S. v. AT&T: Five Lessons for Vertical Merger Enforcement
Oct 30, 2024 by
CPI
The Search for Antitrust Remedies in Tech Leads Beyond Antitrust
Oct 30, 2024 by
CPI